Fast, In-Memory Computing
In-Memory Computing for Operational Intelligence
Operational systems generate streams of fast-changing data that need to be tracked, correlated, and analyzed to identify patterns and trends — and then generate immediate feedback to steer operations. This is called operational intelligence. Organizations that have it can deliver better results, boost cost-effectiveness, and identify perishable business opportunities that others miss. Traditional business intelligence, with its batch processing systems and disk-based data storage, simply cannot keep up with operational systems. In-memory computing can.
ScaleOut StateServer Pro delivers in-memory computing in a form that’s ideal for operational intelligence. It combines ScaleOut’s industry-leading, in-memory data grid with an integrated, data-parallel compute engine to create a breakthrough platform for building applications that generate real-time results. Unlike pure streaming or event processing systems, such as Storm or Spark Streaming, ScaleOut StateServer Pro makes it easy to track and correlate fast-changing data using an in-memory, object-oriented model. Blazingly fast and scalable data-parallel computing identifies important patterns and trends in this data with extremely low latency so that immediate feedback can be generated and action taken. Now operational intelligence is both possible and within easy reach.
Operational Intelligence vs. Business Intelligence
Although business intelligence (BI) has evolved over the last several years with the adoption of Hadoop, its focus remains on examining very large, static data sets to identify long term trends and opportunities. As a result, most BI implementations use latency-insensitive techniques, such as batch processing and disk-based data storage. Although recent innovations, such as Spark, have employed in-memory computing techniques to improve efficiency and lower latency, they are designed to accelerate BI rather than to directly integrate with operational systems.
Until now, operational systems have not been able to deploy computing technology which tracks and analyzes live data to generate immediate feedback, that is, to provide operational intelligence (OI). ScaleOut StateServer Pro was designed to make OI possible. By combining an in-memory data grid with an integrated compute engine, it can track live data with both low latency and high availability using a straightforward, object-oriented model. Now live systems have the technology they need for OI.
Data-Parallel Computing — Made Easy
Data scientists have known for decades that data-parallel computing is both fast and remarkably easier to use than other techniques for parallel processing. Hadoop developers have brought this technology into the 21st century to focus on business intelligence. Now ScaleOut StateServer Pro combines in-memory and data-parallel computing to unlock the benefits of operational intelligence.
ScaleOut StateServer Pro makes data-parallel computing extremely easy for application developers to learn and use by integrating it with popular programming languages such as Java and C#. Called “parallel method invocation” (PMI), this approach lets developers write data-parallel computations as language-defined methods on collections of objects stored in the in-memory data grid. ScaleOut StateServer Pro automatically deploys the code and runs these methods in parallel across the grid. PMI-based applications are simple to write, debug, and maintain — and they run extremely fast.
Unlike complex parallel computing platforms, such as Hadoop and Storm, PMI requires no tuning to extract maximum performance. Its simple, yet powerful data-parallel model derived from parallel supercomputing sidesteps the complexities inherent to Hadoop MapReduce, such as constructing key spaces, optimizing reducers, and combining results. It also avoids Storm’s complex, task-parallel execution pipeline while providing a straightforward means to track incoming events, correlate them, and maintain an in-memory model of a live system.
To make distributed, data-parallel programming accessible to anyone familiar with .NET’s Task Parallel Library (TPL), ScaleOut StateServer Pro includes an operator called “Distributed ForEach” modeled after the TPL’s widely used Parallel.ForEach operator. This feature seamlessly extends data-parallel computing across ScaleOut’s in-memory data grid to handle much larger data sets than otherwise possible. It delivers fast, scalable performance while avoiding network-intensive data motion and unnecessary garbage collection. Integration with LINQ query allows applications to specify exactly which data needs to be processed within a large collection.
Learn more about ScaleOut StateServer Pro’s ease of use.
Integrated Parallel Query
ScaleOut StateServer Pro integrates parallel query into PMI to select objects for analysis, providing a simple, object-oriented filter based on object properties. This can dramatically reduce the processing workload and shorten compute times.
Global Merging of Results
To expedite feeding results back to an operational system, ScaleOut StateServer Pro adds a unique feature to combine results. Developers define a method for merging results, and then PMI runs this method in parallel across all servers to generate a single, globally-combined result.
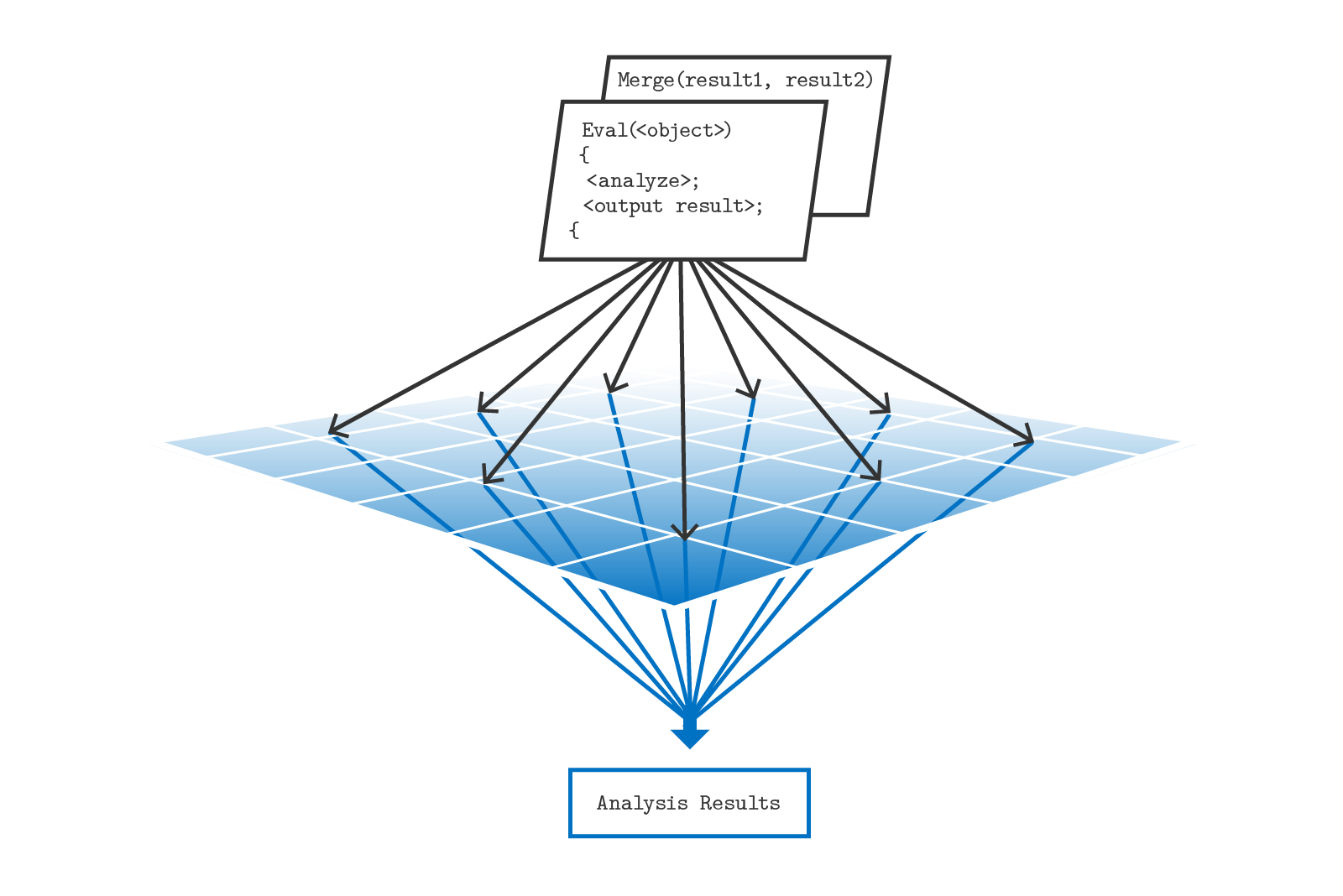
Parallel Method Invocation Runs in Parallel on ScaleOut’s IMDG
Low-Latency and Scalable Performance
When running PMI applications using ScaleOut StateServer Pro, the in-memory data grid’s compute engine automatically squeezes performance out of all grid servers and cores to complete the computation as fast as possible. The engine eliminates batch scheduling overhead, typically starting up computations in less than a millisecond. Since the data is already hosted in memory within the grid, no time is wasted moving data from disk or across the network. PMI delivers the lowest possible latency for performing data-parallel computations and generating operational intelligence.
Operational intelligence needs to be able to maintain low latency even as the system it tracks increases its workload. ScaleOut StateServer Pro makes this easy. Performance scaling simply requires adding servers to the in-memory data grid, which automatically redistributes and rebalances the workload to take advantage of the new servers. Storage capacity, access throughput, and compute power all grow linearly, and execution times stay fast.