In-Memory Power
In-Memory Enables Operational Intelligence
With its scalable performance and ability to analyze petabytes of data, Hadoop has quickly become the standard for business intelligence on huge data sets. However, its batch processing and disk-based data storage have made it unsuitable for use in analyzing live, fast-changing data in production environments to extract important patterns and trends and generate immediate feedback—operational intelligence. Until now.
ScaleOut hServer’s in-memory data storage and integrated compute engine unlock the power of Hadoop for operational intelligence. Instead of storing live fast-changing data on disk within HDFS, ScaleOut hServer uses a fast, scalable in-memory data grid (IMDG) that enables live data to be continuously saved, updated, and analyzed using ScaleOut hServer’s Hadoop MapReduce compute engine. Standard MapReduce applications now can analyze live, fast-changing data with extremely low latency.
Analyze Live Data
ScaleOut hServer’s in-memory data grid stores key/value pairs across an elastic set of networked servers, ensuring fast data access and updates to live data with linear scalability, and high availability using a standard, object-oriented model.
Run MapReduce Unchanged
MapReduce applications use standard Hadoop API libraries; no code changes are needed to run with ScaleOut hServer. Access in-memory data sets or even HDFS files using standard Hadoop input/output formats.
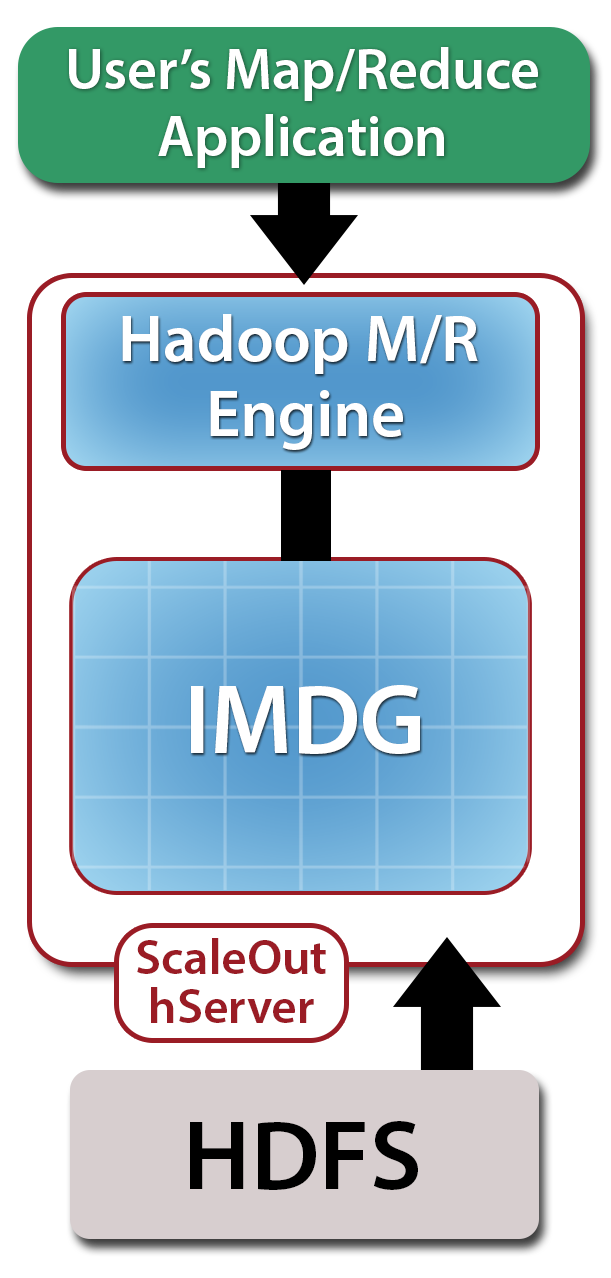
ScaleOut hServer Integrates an In-Memory Data Grid with a MapReduce Compute Engine
Blazingly Fast Execution Times
ScaleOut hServer’s in-memory MapReduce compute engine executes Hadoop MapReduce programs in seconds (or less) by incorporating several techniques not available with standard Hadoop. By avoiding Hadoop’s batch scheduling, it can start up jobs in milliseconds instead of tens of seconds. In-memory data storage dramatically reduces access times by eliminating data motion from disk or across the network. Fast, highly optimized, memory-based storage, combining, data shuffling, and optional sorting further drive down overhead. All of this is accomplished without changing a line of MapReduce code.
Isn’t This Just Spark?
ScaleOut hServer is designed specifically for operational intelligence (OI). Unlike Spark, it uses an in-memory data grid to host fast-changing data. This ensures that memory-based data objects are both individually accessible and highly available. Because Spark was developed to accelerate batch processing, it incorporates a different in-memory data storage model which does not meet the needs of OI.
Benchmarks Demonstrate 40X Speedup
In benchmark testing of a real-world financial services application, ScaleOut hServer demonstrated continuous MapReduce execution at the rate of 350 milliseconds per run in comparison to Apache Hadoop, which clocked in at more than 15 seconds. This application tracks market price changes for a hedge fund to generate alerts when portfolio rebalancing is needed.